Reinforcement learning for life sciences' biggest challenges
At Reliant we develop AI agents that find, organize, and serve answers to the most complex of life science questions – at massive scale. These agents help researchers uncover emerging trends for new therapies, identify patient subgroups with unmet needs, and get evidence-backed estimates of disease prevalence and incidence. In order to support this kind of decision-critical work, our AI agents naturally need to provide answers of the highest quality. Yet in our work we’ve found that both commercial and open-source language models continue to fall short of the accuracy required for our workflows.
To fix this, we use a technique known as reinforcement learning (RL). We use RL to create language models that excel at answering specific questions, for example determining the primary patient population for a given clinical trial. Where traditional AI methods require labelled data, RL improves language models by a process of trial and error, where the model is positively or negatively rewarded based on the answers it proposes and then learns to pick the highest-rewarded answers. Compared to traditional AI methods, RL is advantageous because it lets us obtain feedback quickly: intuitively, it’s easier to check the model’s proposed answer and say, “This isn’t quite right” than to take the time to figure out the correct answer and write it down.
In trying to apply existing RL algorithms to our problem setting, we found that they didn’t perform well when the feedback is both a) mostly negative and b) collected ahead of time (what is called off-policy learning in the field). Unfortunately, this is where most of our interesting data comes from: many of our users’ questions are ambiguous and hard to answer, and collecting learning feedback from domain experts takes time. To address this problem, we created a new algorithm, TOPR, published on arXiv this week. By dealing with negative, off-policy feedback properly, we’re able to use RL to improve language models from a much richer set of data, and consequently make substantial gains in model accuracy.
Beating the benchmarks
On academic benchmarks, we find that TOPR substantially outperforms the gamut of RL techniques that currently form the state-of-the-art in the literature. In particular, we find that TOPR can make the smaller 8-billion parameter Llama 3 language model outperform its larger 70-billion counterpart through the process of reinforcement learning. To understand our algorithm's benefits on a real problem, we used it to train a language model to identify the line of therapy of a clinical trial given its protocol. Doing so is challenging, and experts often disagree on the best answer (for example, whether a trial involves 1L patients only, or 1L+). One of our customers, a Chief of Strategy at a boutique consulting firm, summarizes it well: Existing clinical trial databases and data vendors fail to clearly specify lines of therapy due to inconsistent terminology, unstructured eligibility criteria, and evolving protocols. As a result, their analysis traditionally requires manual interpretation of complex trial descriptions, sometimes leading to confusion and missed opportunities.
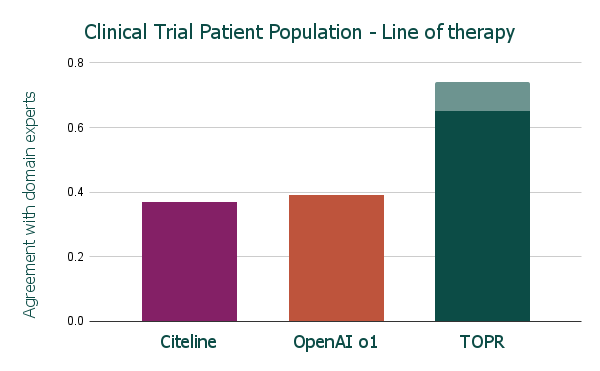
On a carefully-curated benchmark of cancer trials, our domain experts label data from the commercial database Citeline as acceptable only 37% of the time, with OpenAI’s o1 model not much ahead at 39%. By contrast, using just shy of 3000 datapoints, our TOPR-based AI agent achieves 65% agreement with domain experts, with another 9% flagged for review by our validation algorithm. Beyond pure accuracy and data coverage, TOPR is also helping our users quickly gain confidence in their insights, from interactive question answering to smarter source highlighting.
Our users are pioneers in leveraging technology to enhance their workflows. They use Reliant Tabular to provide leading recommendations to commercial strategy teams on market opportunities, assess competitive landscapes, and optimize trial positioning more effectively. Although it’s still early days, there is no doubt that AI and reinforcement learning are here to change how insights are created.